
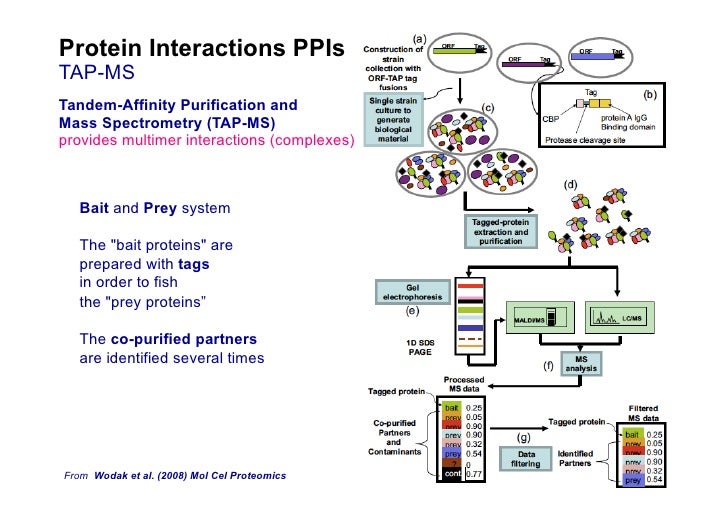
Weber APM (2015) Discovering new biology through RNA-Seq. Martin LBB, Fei Z, Giovannoni JJ, Rose JKC (2013) Catalyzing plant science research with RNA-seq. DNA sequencing costs: data from the NHGRI large-scale genome sequencing program. Malik VS (2016) RNA sequencing as a tool for understanding biological complexity of abiotic stress in plants. Moustafa K, Cross JM (2016) Genetic approaches to study plant responses to environmental stresses: an overview. Usadel B, Fernie AR (2013) The plant transcriptome-from integrating observations to models. Even though the data we used in this example was obtained from Arabidopsis thaliana, the workflow developed in this guide can be easily adapted to work with RNA-seq data from any organism. This guide includes basic instructions for the operation of widely used open source platforms such as Bio-Linux, R, and Cytoscape. Similar strategies have been used in previous studies to advance root developmental biology. In order to make the process of constructing a gene co-expression network more accessible to biologists, here we provide step-by-step instructions using published RNA-seq experimental data obtained from a public database. Many examples of new hypothesis derived from network analyses can be found in the literature, spanning different organisms including plants and specific fields such as root developmental biology. A successful strategy to generate tractable hypotheses from transcriptomics data has been to build undirected network graphs based on patterns of gene co-expression. The opportunity is to use this information to generate testable hypothesis to understand molecular mechanisms controlling gene expression and biological processes (Fig. The challenge is handling, integrating, and interpreting these data sets. The rapid increase in the availability of transcriptomics data generated by RNA sequencing represents both a challenge and an opportunity for biologists without bioinformatics training.
